Security is everybody’s business| Potential AI in Security Services
Do you want to become outstanding using AI Applications in the Security Industry today? If yes, then this article-Unleashing The Potential AI in Security Services is for you. Sit back and read up…
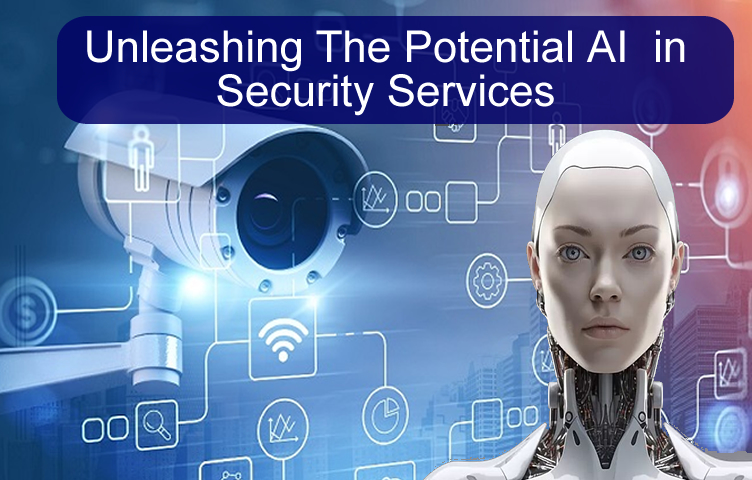
The use of AI-based applications is proliferating in the securities industry and transforming various functions within broker-dealers.
Some large firms have established centers of excellence to review, share, and build expertise and create synergies related to the use of AI across their organizations.
In addition, firms are exploring and incorporating AI tools built by financial technology startups and vendors.
In this section, we highlight three broad areas where broker-dealers are evaluating or using AI in the securities industry: communications with customers, investment processes, and operational functions.
Specifically, with respect to communications with customers, the discussion explores how firms are using AI to enhance customer experience and outreach targeting.
With respect to investment processes, the discussion explores how firms are using AI to assist with brokerage account management, portfolio management, and trading.
Finally, with respect to operational functions, the discussion explores how firms are using AI to assist with compliance, risk management, and administrative tasks.
Furthermore, use of AI applications does not relieve firms of their obligations to comply with all applicable securities laws, rules, and regulations.
AI in Security Services
Communications with Customers
Customer Experience
The use of AI in applications to enhance customer experience has gained significant traction, not just in the securities industry but broadly within the financial services industry.
AI-based customer service applications largely involve NLP- and ML-based tools that automate and customize customer communications.
Virtual assistants: A virtual assistant is an AI application that interacts with humans using voice recognition and synthesized speech and is programmed to perform certain tasks.
Several firms have already deployed, or are in the process of deploying, virtual assistants to provide digital customer service.
Firms have noted that their virtual assistants provide responses to basic customer inquiries, such as those related to account balances, portfolio holdings, market data, address changes, and password resets.
At a few firms, virtual assistants also offer the ability to accept and process trade orders within certain thresholds.
Firms are deploying virtual assistants through different platforms. Several online firms have deployed conversational chatbots through their websites and mobile apps.
16 Some firms noted that they are experimenting with the development of virtual assistant applications available to customers through third-party platforms, such as Amazon’s Alexa, Google’s Home Assistant, and Apple’s Siri.
In addition, some firms are integrating AI-based interactive voice response (IVR) systems at their call centers to respond to basic inquiries from callers or collect sufficient information to facilitate triaging of calls to the appropriate human customer service agents.
These online, device-based, and telephonic virtual assistants employ NLP (including speech-to-text/text-to-speech conversion, tone recognition, and text generation), ML, and sophisticated customer authentication tools, including the use of facial recognition, fingerprints, and voice biometrics.
The applications are trained with large sets of historical and current data, including customer account information, trading history, and past inquiries, as well as market data, to formulate appropriate responses to incoming customer inquiries.
Email inquiries: Some firms indicated that they are using AI-based applications to screen and classify incoming client emails based on key features, such as the sender’s identity, the email’s subject line, and an automated review of the email message itself.
Such applications may also automatically respond to emails containing common or routine inquiries, while routing emails with complex inquiries to the appropriate personnel.
Firms also noted using similar applications to process and triage internal inquiries (e.g., those received by internal help desks), to provide automated responses where possible, and to route more complicated inquiries to relevant subject matter experts.
Outreach Targeting
A number of broker-dealers are exploring the use of AI to target outreach to customers or potential customers.
Some firms are using AI tools to analyze their customers’ investing behaviors, website and mobile app footprints, and past inquiries, and in turn, to proactively provide customized content to them.
This could include curated educational information, news, and research reports on specific investment products or asset classes.
This content could be delivered to customers by email or directly through the firm’s website or mobile app.
In addition, firms have also indicated AI tools are being explored to determine whether individuals would be interested in certain services based on their customer profile and browsing history within the firms’ websites.
Industry participants noted that the exploration and deployment of AI-based applications for customer communications, particularly automated virtual assistants, have gained significant traction because they may help to enhance customer experience, create significant internal cost efficiencies for firms, and provide opportunities for greater engagement between the firm and customer.
However, these applications may also pose certain challenges and potential risks, such as those associated with customer authentication, data privacy, cybersecurity, and recordkeeping.
Investment Processes
Brokerage Account Management
Brokerage account management functions at some large firms are starting to incorporate AI-based tools to provide individual brokers with customized, real-time customer information and better insights into customer preferences and trading behaviors.
Registered representatives use such information to enhance customer relationships, to provide better service and recommendations, and to potentially minimize customer attrition.
The following are some examples shared by firms for the use of AI in understanding customer needs and managing their brokerage accounts:
Holistic customer profiles: Firms are starting to develop AI-based applications that create real-time, holistic customer profiles, which incorporate information from a broad range of sources, such as customer assets (held both at the firm and outside the firm), spending patterns, and debt balances obtained through data aggregation tools; updates on social media and other public websites; browsing history on the firm’s website and mobile apps; and past communications (e.g., from emails, chat messages, and meeting notes).
All this information is analyzed using AI tools to provide the registered representative with a broader picture of customer needs, along with tailored suggestions of what investment products the customer may be interested in.
Industry participants indicated that registered representatives use this information to augment their existing knowledge and expertise when making suggestions to their customers.
Industry participants noted taking a cautious approach to employing AI tools that may offer investment advice and recommendations directly to retail customers, citing several legal, regulatory, and reputational concerns.
Customized research: Firms have also indicated growing use of AI tools to provide curated market research directly to customers to share relevant information on various investment opportunities.
For example, as noted in the earlier section, AI-based tools may offer customers social media data and related sentiment analysis on investment products and asset classes.
While these AI tools offer the potential to customize investment suggestions for customers, firms should be cognizant of potential concerns and challenges related to data privacy, use of corrupt or misleading data, and adapting to each customer’s unique circumstances.
Portfolio Management and Trading
Broker-dealers are also exploring and using AI applications within their portfolio management and trading functions.
Portfolio management: Within portfolio management, firms noted the use of AI applications to identify new patterns and predict potential price movements of specific products or asset classes.
These applications tap into vast amounts of data available from internal and external sources, including from non-traditional sources like social media and satellite imagery, which is used as proxy data for economic activity to identify insights that may signal price movement.
Some broker-dealers that are also investment advisors aim to incorporate these predictions into their investment strategies to generate alpha for the portfolio.
Trading: Securities industry participants are also exploring AI tools to make their trading functions more efficient by maximizing speed and price performance.
Examples include using ML for smart order routing, price optimization, best execution, and optimal allocations of block trades.
Firms should bear in mind that use of AI in portfolio management and trading functions may also pose some unique challenges, particularly where the trading and execution applications are designed to act autonomously.
Circumstances not captured in model training – such as unusual market volatility, natural disasters, pandemics, or geopolitical changes – may create a situation where the AI model no longer produces reliable predictions, and this could trigger undesired trading behavior resulting in negative consequences.
In addition, some industry participants have expressed concern that AI trading models across the industry may start to learn from each other, potentially leading to collusive activity, herd behavior, or unpredictable results.1
Operational Functions
Compliance and Risk Management
In conversations with FINRA staff, industry participants noted that they are spending significant time and resources in developing AI-based applications to enhance their compliance and risk management functions.
This is consistent with FINRA’s 2018 research on the use of regulatory technology (RegTech), where we observed that “market participants are increasingly looking to use RegTech tools to help them develop more effective, efficient, and risk-based compliance programs.
“According to an April 2018 research study conducted by Chartis Research and IBM, which surveyed more than 100 relevant risk and technology professionals, 70% of respondents noted using AI in risk and compliance functions.
Broker-dealers have to keep pace with complex and evolving domestic and international regulations, as well as a rapidly changing risk landscape (e.g., cybersecurity, internal threats, and financial risks).
At the same time, they now have access to vast amounts of data, inexpensive computing power, and innovative technologies that present opportunities for them to develop automated compliance and risk-management tools.
Below are some examples that firms shared of how they are incorporating AI in their compliance and risk management tools.
Surveillance and monitoring: AI technology offers firms the ability to capture and surveil large amounts of structured and unstructured data in various forms (e.g., text, speech, voice, image, and video) from both internal and external sources in order to identify patterns and anomalies.
This enables firms to holistically surveil and monitor various functions across the enterprise, as well as monitor conduct across various individuals (e.g., traders, registered representatives, employees, and customers), in a more efficient, effective, and risk-based manner.
Market participants noted that these tools could significantly reduce the number of false positives, which in turn, free up compliance and supervisory staff time to conduct more thorough reviews of the remaining alerts, resulting in higher escalation rates.
Firms indicate that these tools offer the ability to move beyond “traditional rule-based systems to a predictive, risk-based surveillance model that identifies and exploits patterns in data to inform decision-making.”
For example, some firms noted the use of AI-based surveillance tools to monitor communications with customers across various channels, such as emails, social media, and text messaging.
Firms noted that these tools gave them the ability to move beyond a traditional lexicon-based review to a more risk-based review, such that they could decipher tone, slang, or code words, which may be indicative of potentially risky or non-compliant behavior.
Customer identification and financial crime monitoring: AI-based tools are also being developed for customer identification (also referred to as “know-your-customer” (KYC)) and financial crime monitoring programs, for example, to detect potential money laundering, terrorist financing, bribery, tax evasion, insider trading, market manipulation, and other fraudulent or illegal activities.
Market participants noted that many traditional KYC and financial crime monitoring methods are cumbersome and not as effective as desired, often resulting in high rates of false positives.
Consequently, firms have started incorporating AI technologies, such as ML, NLP, and biometrics, to make their programs more effective and risk based. Firms indicated that these tools enable them to identify and track customer activity with greater accuracy and efficiency, and to conduct more holistic and detailed analysis of customer transactions.
Regulatory intelligence management: Broker-dealers use a variety of regulatory intelligence management programs and processes to identify, interpret, and comply with new and changing rules and regulations across jurisdictions.
While this has traditionally been a manual process, firms are now exploring the use of AI tools to digitize, review, and interpret new and existing regulatory intelligence (including rules, regulations, enforcement actions, and no-action letters) and to incorporate appropriate changes into their compliance programs.
Some industry participants noted that automated regulatory intelligence management programs have the potential to increase overall compliance, while reducing both costs and time spent implementing regulatory change.
According to a research report that explores the use of AI by financial institutions for risk and compliance functions, “automating the process of regulatory change management is something of a ‘holy grail’ in the use of AI.”Some regulators are also exploring and adopting the concept of “machine-readable” rulebooks, which could potentially enable firms to automate the process of identifying, categorizing, and mapping the rules to relevant regulatory obligations within their internal workflows.
Liquidity and cash management: In our discussions, some firms noted that they are employing ML applications to optimize their financial liquidity and cash management.
Such applications analyze substantial historical data along with current market data to identify trends, note anomalies, and make predictions, for example, related to intra-day liquidity needs, peak liquidity demands, working capital requirements, and securities lending demand.
Credit risk management: Broker-dealer firms are also employing AI-based models to assess creditworthiness of their counterparties, which both speeds up the credit review process and allows the incorporation of non-traditional criteria (e.g., information available through social media).
However, some AI-based credit-scoring systems have faced criticism for being opaque and potentially biased and discriminatory.
These models not only analyze traditional credit-evaluation criteria, such as current financial standing and historical credit history, but may also identify other demographic factors as deterministic criteria, which could lead to unfair and discriminatory credit scoring based on biases present in the underlying historical data. (Refer to the Key Challenges and Regulatory Considerations for additional discussion on the topic.)
Cybersecurity: Cybersecurity continues to be a top challenge for the financial services industry. Perpetrators are continuously evolving and using sophisticated technology, including AI, to conduct their attacks.
In addition, regulators are requiring financial institutions to develop comprehensive cybersecurity controls. In response, broker-dealers are starting to incorporate AI as an essential component of their cybersecurity programs.
A recent research report noted that “sixty-nine percent of organizations believe AI will be necessary to respond to cyberattacks.” Incorporating AI into cybersecurity programs may allow firms to assist overwhelmed cybersecurity staff to predict potential attacks, detect threats in real-time, and respond to them faster and at lower costs.
Use of AI in cybersecurity programs often begins within insider risk programs where normal behavior can be learned and then deviations or anomalies can be flagged as a risk and reviewed.
Administrative Functions
Another area where AI is being widely used by the securities industry is the automation of administrative functions. Firms are incorporating software augmented by AI technologies (e.g., ML, NLP, and CV) to automate high-volume, less complex, repetitive, and manual tasks that traditionally involve significant human staff time.
Firms indicated that automating such tasks with AI tools have the potential of high returns in terms of cost savings and efficiency increases. The following are select examples shared by some broker-dealers of AI use cases for administrative functions:
Automation of manual, paper-based processing: Some firms have deployed AI tools to automate functions that involve manual review of documents, such as processing faxed trade orders, depositing physical checks, and searching, ranking, and retrieving documents.
These applications incorporate CV and NLP to identify customers, review signatures, read orders, and digitize documents.
Firms indicated that such applications not only increase productivity, but also accelerate important processes, such as trade and payment processing.
Document review and information extraction: NLP enables firms to review significant volumes of documents (e.g., legal contracts, custody documents, and loan agreements) at a fraction of the time it takes for human analysis.
In addition to time and cost savings, some firms have indicated that these automated reviews have yielded results with higher accuracy.
For example, some firms noted that they use NLP and unsupervised ML to review and classify legal contracts, or to look for certain clauses or key data points within a category of contracts.
Another firm noted the use of AI to automate the review of prospectuses for key information, such as expense ratios.
Other applications: Industry participants also indicated they are exploring other areas related to operations where AI tools help to improve the accuracy and efficiency of existing processes, such as reporting and invoice reconciliation.
Potential AI in Security Services